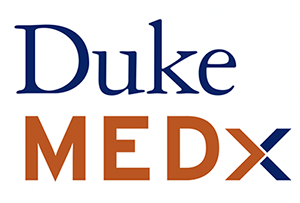
MEDx grant opportunities are one mechanism to encourage and support new collaborations or expansion of existing collaborations between faculty in the Schools of Medicine and Engineering. With the most recent request for proposals, MEDx invited faculty to submit proposals that they considered to be high-risk – unlikely to be funded through other mechanisms without preliminary data – but potentially highly impactful to medical treatment or the preservation of health.
This cycle, MEDx particularly encouraged teams, composed of early-stage investigators, to apply for funding.
MEDx received 10 very worthy applications exploring a wide range of topics and exhibiting a high degree of novelty. MEDx awarded grants to three projects that will be tracked over the course of the performance year and after. We look forward to reporting on each team’s successes in the future.
Sincere thanks to the many reviewers who volunteered precious time to read the applications and provide thoughtful feedback.
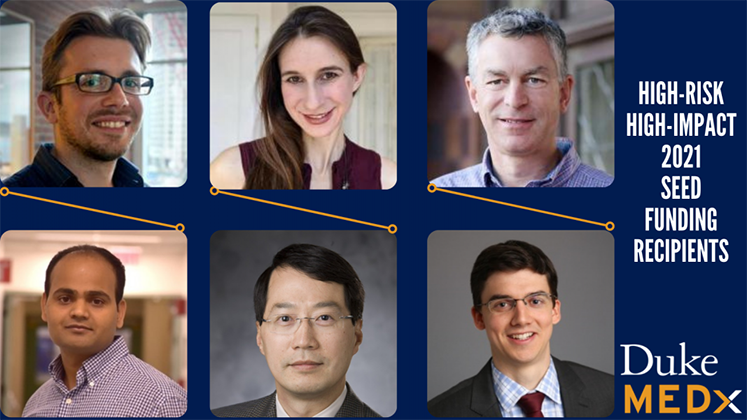
Congratulations to the 2021 recipients!
IntraVascular Membrane Oxygenator Whole Blood Proof of Concept
Marc Deshusses, PhD | Professor of Civil and Environmental Engineering
Tobias Straube, MD | Assistant Professor of Pediatrics
Acute Respiratory Distress Syndrome, a form of acute lung injury resulting in hypoxic respiratory failure is a frequent and often lethal indication for admission to both adult and pediatric intensive care units. The incidence of this syndrome has increased with the COVID-19 pandemic. With the pandemic, the general public has become familiar with the use of mechanical ventilation to improve oxygenation for patients. However, when the lungs are so compromised that mechanical ventilation fails to relieve patients’ hypoxia, clinicians may turn to veno-venous extracorporeal membrane oxygenation, or ECMO. Unfortunately, ECMO is associated with several potentially serious complications and is a complex treatment available in a limited number of hospitals. To address these problems, the team led by Deshusses and Straube aims to develop an intravascular oxygenation catheter that will deliver clinically significant amounts of oxygen to patients with hypoxic respiratory failure independent of their lung function.
AI-driven drug discovery of P2ry14 antagonists against pulmonary fibrosisDaniel Reker, ScD | Assistant Professor of Biomedical Engineering
Daniel Reker, ScD | Assistant Professor of Biomedical Engineering
Purushothama Rao Tata, PhD | Assistant Professor of Cell Biology and Medicine (Pulmonary, Allergy, and Critical Care)
The team led by early career investigators Daniel Reker and Purushothama Rao Tata, is capitalizing upon earlier, single cell transcriptome studies, which identified the P2ry14 puroginergic receptor as a significant player in various inflammatory diseases including idiopathic pulmonary fibrosis. Idiopathic pulmonary fibrosis is a devastating condition for which there are few treatments and none that stop disease progression. Tata and Reker hypothesize that P2RY14 is a potential therapeutic target for pulmonary fibrosis. However, there is no clinically approved drug for this receptor. By combining artificial intelligence -driven drug discovery approaches used in Reker’s lab with novel experimental models developed by Tata, the team aims to rapidly discover and functionally validate designer drugs targeted to P2RY14. Their research will also prototype the integration of experimental biology and deep active machine learning for drug discovery.
Interpretable Deep Learning Models for Better Clinician-AI Communication in Clinical Mammography
Cynthia Rudin, PhD | Professor of Computer Science, Electrical and Computer Engineering, Statistical Science, and Biostatistics & Bioinformatics
Joseph Lo, PhD | Professor and Vice Chair of Research for Radiology
Deep learning technologies are now pervasive in radiology, but those models approved by the FDA are black boxes that deliver results that clinicians may not fully understand and therefore may not trust. At least in part because of this lack of understanding for what drives these black box, deep learning tools to deliver a particular result, there has been slow adoption of these assistive technologies. Rudin and Lo believe that accurate AI models for radiology do not need to be black boxes. Prior work by this team shows that it is possible for AI to explain its reasoning processes in an interpretable way that humans can understand. Their goal is to change the current paradigm for breast cancer diagnosis. The project leverages advances in computer vision safely, while leaving power and responsibility in the hands of trained clinicians. If successful, this project has the potential to reduce the number of unnecessary breast biopsies and improve the quality and consistency of breast cancer screening. Further, successful implementation of an interpretable model to aid breast screening may be important for the adoption of other AI technologies in medicine.